Federated learning is a double-edged sword in that it is designed to ensure data privacy, yet unfortunately, it opens a door for adversaries to exploit the system easily. One of the popular attack vectors is a poisoning attack.
What is a poisoning attack?
A poisoning attack aims to degrade machine learning models easily that can be classified into two categories: data and model poisoning attacks.
Data poisoning attacks aim to contaminate the training data to indirectly degrade the performance of machine learning models [1]. Data poisoning attacks can be broadly classified into two categories: (1) label flipping attacks in which an attacker "flips" labels of training data [2] (2) backdoor attacks in which an attacker injects new or manipulated training data, resulting in misclassification during inference time [3]. An attacker may perform global or targeted data poisoning attacks. Targeted attacks add more challenges to be detected as they only manipulate a specific class and make data for other classes intact.
On the other hand, a model poisoning attack aims to directly manipulate local models to compromise the accuracy of global models. Model poisoning attacks can be performed as untargeted or targeted attacks similar to data poisoning attacks. An untargeted attack aims to degrade the overall performance and achieve a denial of service. A targeted attack is a more sophisticated way to corrupt model updates for subtasks while maintaining high accuracy on global objectives [1].
A federated learning system is inherently vulnerable to poisoning attacks due to its inaccessibility to local data and models in individual participants. Targeted attacks make the problem worse as it would be extremely hard for a central server to identify attacks in the models given high accuracy on global objectives.
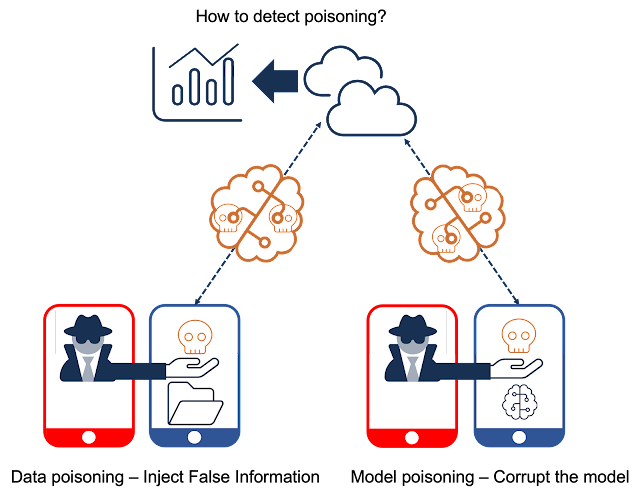
How can we defend?
Approaches to defend against poisoning attacks can be classified into two categories: (1) Robust Aggregation and (2) Anomaly Detection. A typical aggregation method in the federated learning system is the average of local models to get a global model. For example, each client computes gradients in each round of training phases, the gradients are sent to a central server, and the server computes the average gradients in FedSGD [4]. For better efficiency, each client computes the gradient and updates the model for multiple batches, and the model parameters are sent to the server, and the server computes the average of the model parameters in FedAvg [5]. Apparently, these average-based approaches will be susceptible to poisoning attacks. The research focus has been thus on how to better aggregate the model parameters while minimizing such as median aggregation [6], trimmed mean aggregation [6], Krum aggregation [7], or adaptive averaging algorithms [1].
A more proactive way to defend against poisoning attacks is filtering malicious updates through anomaly detection. Model updates of malicious clients are often distinguishable from those of honest clients. One defense method is clustering-based approaches that check model updates at the central server, cluster model updates, and filter suspicious clusters from aggregation [8]. Behavior-based defense methods measure differences in model updates between clients and filter malicious model updates from aggregation [8].
Conclusion
A federated learning system has recently emerged and thus the research on attacks against it is still in its early stage. To fully take advantage of the promising potentials of the federated learning system, a lot of research efforts are needed to provide robustness against poisoning attacks.
References
- [1] Jere, Malhar S., Tyler Farnan, and Farinaz Koushanfar. "A taxonomy of attacks on federated learning." IEEE Security & Privacy 19, no. 2 (2020): 20-28.
- [2] Tolpegin, V., Truex, S., Gursoy, M. E., & Liu, L. (2020, September). Data poisoning attacks against federated learning systems. In European Symposium on Research in Computer Security (pp. 480-501). Springer, Cham.
- [3] Severi G, Meyer J, Coull S, Oprea A. {Explanation-Guided} Backdoor Poisoning Attacks Against Malware Classifiers. In30th USENIX Security Symposium (USENIX Security 21) 2021 (pp. 1487-1504)
- [4] Mhaisen, Naram, Alaa Awad Abdellatif, Amr Mohamed, Aiman Erbad, and Mohsen Guizani. "Optimal user-edge assignment in hierarchical federated learning based on statistical properties and network topology constraints." IEEE Transactions on Network Science and Engineering 9, no. 1 (2021): 55-66
- [5] Zhou, Yuhao, Qing Ye, and Jiancheng Lv. "Communication-efficient federated learning with compensated overlap-fedavg." IEEE Transactions on Parallel and Distributed Systems 33, no. 1 (2021): 192-205
- [6] Yin, D., Chen, Y., Kannan, R., & Bartlett, P. (2018, July). Byzantine-robust distributed learning: Towards optimal statistical rates. In International Conference on Machine Learning (pp. 5650-5659). PMLR.
- [7] Blanchard, Peva, El Mahdi El Mhamdi, Rachid Guerraoui, and Julien Stainer. "Machine learning with adversaries: Byzantine tolerant gradient descent." Advances in Neural Information Processing Systems 30 (2017)
- [8]Awan, Sana, Bo Luo, and Fengjun Li. "Contra: Defending against poisoning attacks in federated learning." In European Symposium on Research in Computer Security, pp. 455-475. Springer, Cham, 2021
- [9] Fang, Minghong, Xiaoyu Cao, Jinyuan Jia, and Neil Gong. "Local Model Poisoning Attacks to {Byzantine-Robust} Federated Learning." In 29th USENIX Security Symposium (USENIX Security 20), pp. 1605-1622. 2020
About Scalytics
Scalytics Connect is a next-generation Federated Learning Framework built for enterprises. It bridges the gap between decentralized data and scalable AI, enabling seamless integration across diverse sources while prioritizing compliance, data privacy, and transparency.
Our mission is to empower developers and decision-makers with a framework that removes the barriers of traditional infrastructure. With Scalytics Connect, you can build scalable, explainable AI systems that keep your organization ahead of the curve. Break free from limitations and unlock the full potential of your AI projects.
Apache Wayang: The Leading Java-Based Federated Learning Framework
Scalytics is powered by Apache Wayang, and we're proud to support the project. You can check out their public GitHub repo right here. If you're enjoying our software, show your love and support - a star ⭐ would mean a lot!
If you need professional support from our team of industry leading experts, you can always reach out to us via Slack or Email.